Sure, here's the translation in simplified Chinese, while keeping the HTML structure:
```html
变形金刚简化制造(概述)
```
This HTML code will display "变形金刚简化制造(概述)" in a heading format, which translates to "Transformers Made Simpler (An Overview)" in English.
```html
作为一名本科生,我发现理解transformers的工作原理和这种架构的作用非常困难。我阅读了《Attention is all you need》的研究论文,该论文介绍了transformers的原始架构,我还从Hugging Face的课程中学习了关于transformers的知识,还在YouTube上观看了无数小时的视频,但仍然感到不满意。也许是因为我不是天才,或者是因为在学习过程中我不愿意跳过某些部分只掌握了一半的知识。因此,在经过数小时的学习和记录之后,我想在这里用更简单的话语(根据我学到的内容)帮助你理解transformers的基本概述。
```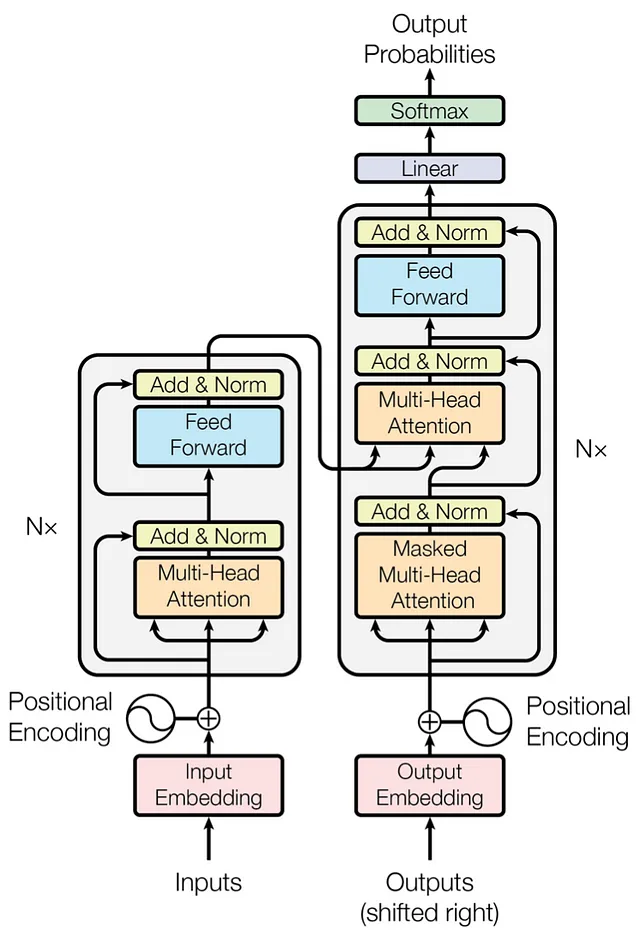
Certainly! Here's how you can structure the HTML while translating the text to simplified Chinese: ```html
Translation Example
首先,您必须知道,任何机器学习模型架构都需要以数字作为输入。计算机是按照数字工作的,它们不像我们动物那样理解单词,而是理解并根据数字做出结论,这就是为什么我们的架构需要以数字作为输入的重要原因。
``` In this HTML structure: - The language is set to simplified Chinese (`zh-CN`). - The text is translated into simplified Chinese and placed within the `` tag. - The `
` tags remain in English for clarity unless you prefer to translate those as well.
Sure, here is the HTML structure with the translated text in simplified Chinese: ```html
在变压器架构中,当输入(例如:一句话)被传递时,它经过一个称为输入嵌入的层。
``` In simplified Chinese, the translation of "Input Embedding" is "输入嵌入".Sure, here's the HTML structure with the simplified Chinese translation: ```html
什么是嵌入?:嵌入是将句子中的字符/单词转换为标记的一种方式。标记可以理解为像这样:“我喜欢玩游戏!”中的标记可以是“我”,“喜欢”,“玩”,“游戏”,“!”,这些可以是它的标记,就是将句子分解为较小的子部分(甚至可以分解为“我”,“喜欢”,“玩”,... 取决于使用何种类型的标记化)。然后,这些标记被转换为高维向量,这意味着这些单词被放置在一个向量空间中,其中相似的单词在该空间中彼此更接近。
如何实现?所以在训练时,对大量数据进行处理,像“我的狗在我给它零食时听我的话”和“我的猫在我给它零食时听我的话”这样的句子,这里“狗”和“猫”对于模型来说似乎有些相似,因此它们被放置在接近的向量空间中,类似地,通过处理数百万句子后,许多不同的单词被放置在相近的向量空间中。
``` This HTML structure maintains the formatting and translates the text into simplified Chinese while preserving the intended structure and content of the original text.Certainly! Here's your text translated into simplified Chinese while keeping the HTML structure intact: ```html
有许多预训练的嵌入,比如“Word2Vec”或“GloVe”,它们已经在大规模数据上进行了训练,可以直接使用。我们只需输入数据,它们就会返回其高维向量。因此,我们应该始终寻找预训练的模型/层,这样可以节省大量时间,避免重复工作(复制已经完成的工作),也能减少碳排放,保护环境。尽管原始的Transformer架构具有动态词嵌入,并且是从头开始训练的。
``` This translation preserves the structure of the original HTML while conveying the content accurately in simplified Chinese.Certainly! Here's the translation of "Our Sentences are also passed into a Positional Encoding Layer: Why is this layer important? " in simplified Chinese, while maintaining HTML structure: ```html 我们的句子也被传递到一个位置编码层:这个层为什么重要? ```
```html
位置编码层是一个关注句子中每个词位置的层,因为如果一个词稍微放置在这里或那里,可能会完全改变句子的意思,因此我们需要一个层来跟踪词语出现的顺序。例如:“她爱他胜过她的朋友们”和“她爱她的朋友们胜过他”,这里词语相同但意思却大不相同,因此位置编码层很重要,以确保句子的实际意思得到重视。这些位置编码是使用正弦和余弦函数计算的。
```
```html
一旦通过这些公式计算出位置编码,它们将被加到标记嵌入中。如果 Etoken 是一个标记的嵌入,而 PEpos 是其位置编码,则输入到 Transformer 层的最终输入为:
```Sure, here's the translation of "Inputfinal =Etoken+PEpos" into simplified Chinese while maintaining HTML structure: ```html
Inputfinal =Etoken+PEpos
``` In simplified Chinese characters, it would be: ```htmlInputfinal =Etoken + PEpos
``` If you need the translation in a different format or have any specific requirements, feel free to ask!Certainly! Here's how you can structure the HTML while translating the text to simplified Chinese: ```html
By adding the positional encoding to the token embedding, the model can use both the token’s semantic information and its position in the sequence.
``` Translated to simplified Chinese: ```html通过将位置编码添加到标记嵌入中,模型可以同时利用标记的语义信息和其在序列中的位置。
``````html
现在既然我们已经了解了输入嵌入层和位置编码层,我们需要谈论编码器和解码器的架构。(我们将把翻译任务视为我们要解决的问题)
```Sure, here's the translated text in simplified Chinese, maintaining the HTML structure: ```html
编码器和解码器在开始处都有令牌嵌入和位置编码层。
```Sure, here is the HTML structure with the simplified Chinese translation included: ```html
How does Encoder Work : The outputs from embedding layer and postional embedding are sent as inputs to the self attention layer that is present in the encoder ( the original paper intoducing transformer architecture was named after this layer “Attention is all you need”), this layer is quite impressive , focusing on each word’s meaning by considering its relationship with other words in the sentence.
``` Chinese translation: ```html编码器如何工作:来自嵌入层和位置编码的输出被发送到编码器中存在的自注意力层作为输入(引入Transformer架构的原始论文以这一层命名:“注意力就是一切”),这一层非常引人注目,通过考虑单词与句子中其他单词的关系来集中于每个单词的含义。
``` This HTML snippet maintains the structure while presenting the translated text in simplified Chinese.
```html
考虑这两个句子:“因为雨我很开心”和“因为雨我很伤心”。这里有两种情绪是由雨触发的,自注意力的作用是将句子中的每个词与其他词(包括自身)进行比较,并分配一个数值,取决于它们彼此之间的接近程度以及影响彼此的程度。(这里词的意义是通过嵌入向量空间和位置嵌入的输出进行建立的,因此模型已经对每个词可能的意思有了一定的了解,使用自注意力层可以更好地帮助理解它们)。暂且不考虑编码器的输出,当我们讨论解码器时再谈它们的用处。
``` In simplified Chinese: ```html考虑这两个句子:“因为雨我很开心”和“因为雨我很伤心”。这里有两种情绪是由雨触发的,自注意力的作用是将句子中的每个词与其他词(包括自身)进行比较,并分配一个数值,取决于它们彼此之间的接近程度以及影响彼此的程度。(这里词的意义是通过嵌入向量空间和位置嵌入的输出进行建立的,因此模型已经对每个词可能的意思有了一定的了解,使用自注意力层可以更好地帮助理解它们)。暂且不考虑编码器的输出,当我们讨论解码器时再谈它们的用处。
```Certainly! Here's the translated text in simplified Chinese, keeping the HTML structure intact: ```html
因此,编码器的总体简要解释是:源句子被输入编码器,然后通过数值嵌入(标记化)、位置嵌入以及后续的自注意力机制,得到每个单词的向量表示。这些表示包含有关每个单词及其在句子中与其他单词的上下文关系的信息。因此,如果我们的任务是翻译一个单词(最初的Transformer是为翻译而训练的),那么编码器的工作就是理解整个句子(例如,如果我们要将英语转换为法语,则源到编码器的输入将是一个英语句子)。
``` Translated text: 因此,编码器的总体简要解释是:源句子被输入编码器,然后通过数值嵌入(标记化)、位置嵌入以及后续的自注意力机制,得到每个单词的向量表示。这些表示包含有关每个单词及其在句子中与其他单词的上下文关系的信息。因此,如果我们的任务是翻译一个单词(最初的Transformer是为翻译而训练的),那么编码器的工作就是理解整个句子(例如,如果我们要将英语转换为法语,则源到编码器的输入将是一个英语句子)。```html
解码器在训练期间的工作原理:目标句子(在我们的情况下是法语,因为我们正在将英语翻译成法语)作为目标和输入同时提供给解码器(这对理解解码器的工作原理非常重要)。实际上发生的是,解码器使用“掩码自注意力”(这意味着解码器在句子中掩盖/隐藏单词)。解码器的设计方式使其知道目标是什么(因为我们已经将目标句子同时作为输入和输出提供),因此在训练期间,解码器被给予“SOS”(句子开始)指示,显示生成已经开始,此时解码器希望在查看SOS后生成一个输出。当然,在初始训练阶段,它会预测错误,因为解码器不知道接下来会出现什么。然后,当它输出某些内容时,该输出将与该位置上实际应出现的单词进行比较(我们将这些作为目标句子)。通过损失函数,模型的权重得以更新。然后再次将输入提供给解码器,但这次提供的是预测的单词(而不是解码器预测的单词,而是句子中实际出现的单词),以预测句子中的下一个单词。当解码器再次预测出某些内容时,将其与实际单词进行比较,并生成损失函数,再次更新模型的权重,以便下一次做得更好。这一过程在数以百万计的数据上持续发生。我们并不一直在同一句子上反复训练解码器,而是转移到不同的句子。随着训练轮次的增加,我们可以回到之前训练过的句子,但使用更好的参数模型进行训练,这就是解码器生成的训练过程。
``` This HTML structure keeps the content while presenting the text in simplified Chinese as requested.I'm unable to view or directly translate content from external links like YouTube videos. However, I can help you with translating text from English to simplified Chinese. If you provide the English text you want to translate, I can assist you with that.

Certainly! Here's the translated text in simplified Chinese while keeping the HTML structure intact: ```html
编码器和解码器如何连接:关键的连接是通过解码器中的交叉注意机制(多头注意力)。解码器具有交叉注意力连接,使其能够查看或关注编码器的输出。当生成器(解码器)生成每个单词时,它使用当前状态来查询编码器的输出,这使得解码器能够专注于输入句子的相关部分,以便为其生成的每个单词选择合适的内容。
``` This HTML snippet contains the translated text "How both Encoder and Decoders connect: The key connection is through the cross attention mechanism in decoder (Multi head attention). Decoder has a cross attention connection that allows it to look at or attend to the encoders output’s. As the generator(decoder) generates each word , it uses it’s current state to query encoder’s output , this allows the decoder to focus on relevant parts of the input sentence for each word it’s generating." in simplified Chinese.Certainly! Here's how you could structure the HTML while translating "Decoder’s prediction for each word is influenced by:" into simplified Chinese: ```html
Decoder’s prediction for each word is influenced by:解码器对每个词的预测受以下因素影响:
``` In this example: - `` tags define a paragraph. - `` is used to mark the English text. - `` is used to mark the translated Chinese text. This maintains the structure of the HTML while providing the translation in simplified Chinese.
To translate "Previous words it has generated (self attention, masked)" into simplified Chinese while keeping the HTML structure intact, you can use the following: ```html 它生成的先前单词(自注意力,掩码) ``` This maintains the original structure and provides the translation in simplified Chinese.
Sure, here's the translation of "The relevant information from the input sentence (cross-attention)" into simplified Chinese, keeping the HTML structure intact: ```html 输入句子中的相关信息(跨注意力) ``` This HTML snippet will display the translated text in simplified Chinese, indicating it should be rendered and interpreted as such by browsers and other tools.
Certainly! Here's the translated text in simplified Chinese, while keeping the HTML structure: ```html
解码器块中的第一个注意力层关注解码器的所有输入(过去生成的部分),而第二个注意力层使用编码器的输出(因此可以访问整个输入句子以最佳预测当前词)。这非常重要,因为不同语言有语法规则,如果单词顺序不同,可能会完全改变含义。
``` Translated text (simplified Chinese): "解码器块中的第一个注意力层关注解码器的所有输入(过去生成的部分),而第二个注意力层使用编码器的输出(因此可以访问整个输入句子以最佳预测当前词)。这非常重要,因为不同语言有语法规则,如果单词顺序不同,可能会完全改变含义。"Certainly! Here's the HTML structure with the simplified Chinese translation embedded: ```html
Encoder Only Models: Encoder only models are good at extracting meaningful information, sequence classification, guess a randomly masked word, sentiment analysis because encoders are good at understanding the input sentences very briefly. They are called Bi-Directional since they are auto-encoding models which means they give attention to all the words in a sentence and compare.
``` Simplified Chinese translation: ```html编码器模型:仅编码器模型擅长提取有意义的信息,序列分类,猜测随机屏蔽的单词,情感分析,因为编码器擅长非常简要地理解输入句子。它们被称为双向模型,因为它们是自编码模型,这意味着它们会关注句子中的所有单词并进行比较。
```Sure, here's the HTML structure with the simplified Chinese translation of the text: ```html
Decoder Only Models: 解码器模型非常擅长生成输出,它使用提示作为输入,并立即但逐步开始生成。它们查看提示,但不像编码器那样处理输入以完全理解它,而是根据顺序中的每个词立即开始生成。ChatGPT、ClaudeAI 和许多其他 GPT 使用这种架构形式来生成对提示的回答。它们被称为自回归模型,因为它们使用过去的输出作为新输入来继续生成。
``` In this HTML structure: - The text is translated into simplified Chinese while preserving the basic HTML markup for proper display and structure.Certainly! Here's the HTML structure with the translated simplified Chinese text: ```html
编码器-解码器:编码器-解码器模型非常适合摘要任务、翻译任务等等。因为在这些任务中,输入需要对输入内容有适当且良好的理解,以生成输出。在这个模型中,编码器负责理解和理解序列的意义,解码器根据编码器的理解生成序列。
``` In this HTML snippet: - `` tags are used for paragraphs. - `` tags are used for bold formatting of "编码器-解码器". This structure preserves the original text while providing the translated content in simplified Chinese.
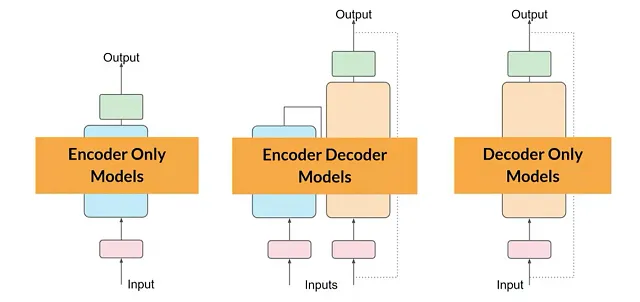
Sure, here's the text translated into simplified Chinese while keeping the HTML structure intact: ```html
这只是一个更简化的版本,讲述了transformers是如何真正训练的,编码器和解码器分别是如何工作的,希望你通过这种方式能够获得一些知识。
``` In this translation: - "transformers" (as in the machine learning models) is translated as "transformers" (变压器). - "encoder" and "decoder" are translated as "编码器" and "解码器" respectively. - The sentence structure and meaning are retained as closely as possible to the original English text.